AI-Powered Basketball Action Recognition: Enhancing C3D with Dynamic Residual Attention
DOI:
https://doi.org/10.70695/HBIB4495摘要
Aiming at the problem that the features extracted from the original C3D (Convolutional 3D) convolutional neural network(C3D) were insufficient and it was difficult to focus on keyframes, which led to the low accuracy of basketball players' action recognition, a basketball action recognition method of deep neural network based on dynamic residual attention mechanism was proposed. Firstly, the traditional C3D is improved to a dynamic residual convolution network to extract sufficient feature information. Secondly, the extracted feature information is selected by the improved attention mechanism to obtain the key video frames. Finally, the algorithm is compared with the traditional C3D in order to demonstrate the advance and applicability of the algorithm. Experimental results show that this method can effectively recognize basketball posture, and the average accuracy of posture recognition is more than 97%.
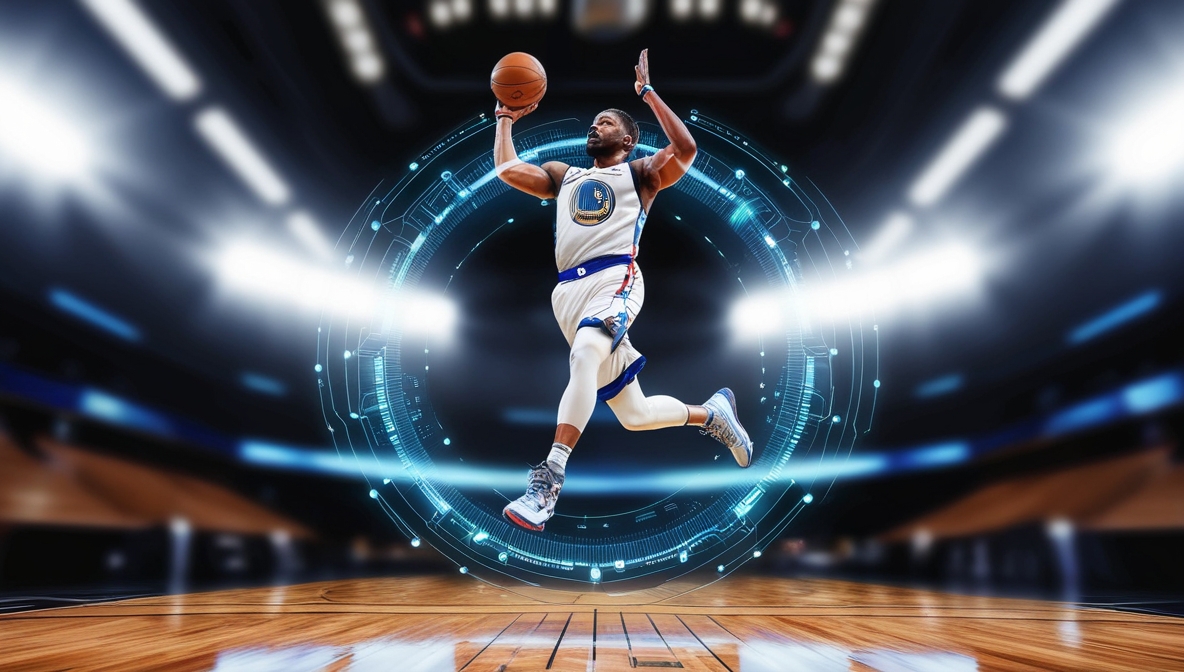